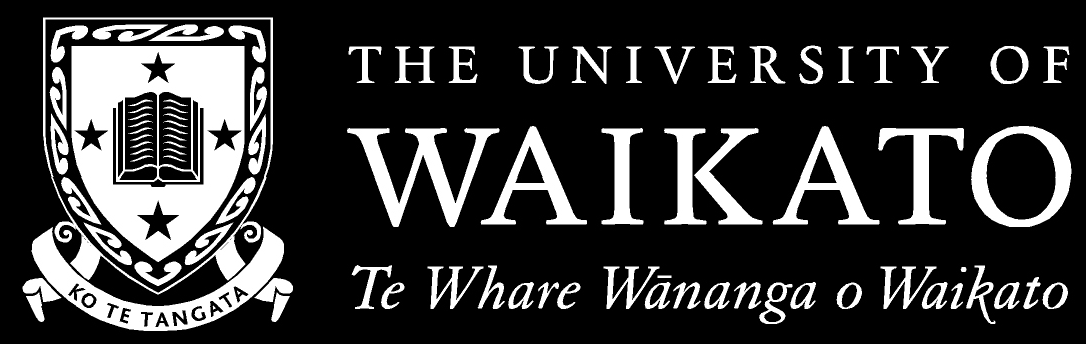
COMPX310-23B (TGA)
Machine Learning
15 Points
Edit Header Content
The University of Waikato
Academic Divisions
Division of Health Engineering Computing & Science
School of Computing and Mathematical Sciences Office
Department of Computer Science
Staff
Edit Staff Content
Convenor(s)
Bernhard Pfahringer
bernhard.pfahringer@waikato.ac.nz
|
|
Nick Lim
9011
FG.2.07
nick.lim@waikato.ac.nz
|
|
Administrator(s)
: buddhika.subasinghe@waikato.ac.nz
Librarian(s)
: anne.ferrier-watson@waikato.ac.nz
You can contact staff by:
- Calling +64 7 838 4466 select option 1, then enter the extension.
-
Extensions starting with 4, 5, 9 or 3 can also be direct dialled:
- For extensions starting with 4: dial +64 7 838 extension.
- For extensions starting with 5: dial +64 7 858 extension.
- For extensions starting with 9: dial +64 7 837 extension.
- For extensions starting with 3: dial +64 7 2620 + the last 3 digits of the extension e.g. 3123 = +64 7 262 0123.
Edit Staff Content
What this paper is about
Edit What this paper is about Content
This paper introduces Machine Learning which is the science of making predictions. ML algorithms strive to be fast and highly accurate, while processing large datasets. This paper will use standard Python-based ML toolkits to teach the fundamentals of ML.
Edit What this paper is about Content
How this paper will be taught
Edit How this paper will be taught Content
Two lectures per week, that are live-streamed on Zoom, and recorded, plus a weekly assignment that you can get help with in the lab or on Zoom, at specific hours.
Edit How this paper will be taught Content
Required Readings
Edit Required Readings Content
Edit Required Readings Content
Learning Outcomes
Edit Learning Outcomes Content
Students who successfully complete the course should be able to:
Edit Learning Outcomes Content
Edit Learning Outcomes Content
Assessments
Edit Assessments Content
How you will be assessed
Edit How you will be assessed Content
Please note that all assessment dates and times below are subject to change. The Moodle/elearn settings will be the correct ones. Make sure to check them.
If you are enrolled in a BE(Hons), samples of your work may be required as part of the Engineering New Zealand accreditation process for BE(Hons) degrees. Any samples taken will have the student name and ID redacted. If you do not want samples of your work collected then please email the engineering administrator, Natalie Shaw (natalie.shaw@waikato.ac.nz), to opt out.
Edit How you will be assessed Content
The internal assessment/exam ratio (as stated in the University Calendar) is 100:0. There is no final exam.
Edit Assessments Content